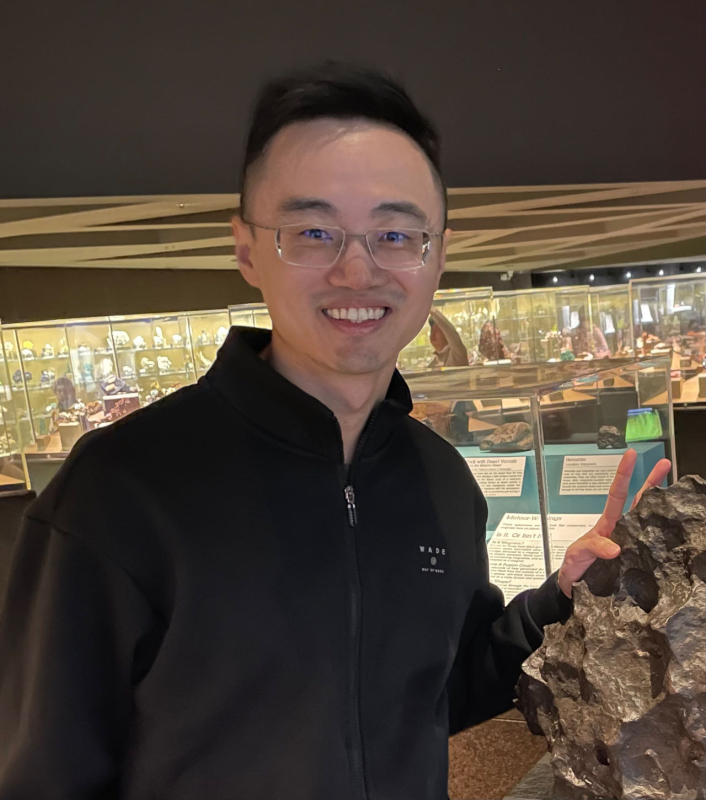
Dr. Kailong Li’s research focuses on hydrological modeling and agricultural water resources allocation. His current work involves developing physics-guided deep learning models to predict and understand post-wildfire hydrological processes.
Before joining DRI, Dr. Kailong earned his Ph.D. in Environmental Systems Engineering from the University of Regina, Canada. He has also been a postdoctoral fellow at the Global Institute for Water Security (GIWS) at the University of Saskatchewan. His past research includes (a) bridging the gap between process-based hydrological models and machine learning, (b) using interpretable machine learning to gain insights into runoff-generation mechanisms, (c) joint-probabilistic extreme flood simulation under changing climatic conditions, and (d) water resources optimization for irrigated watersheds.
Research Areas of Interest
- Deep Learning-Based Large-scale Hydrological Modeling and Inferences
- Interpretable Machine Learning
- Post-Wildfire Hydrological Processes
- Process-Based Hydrological Modeling
- Agricultural Water Resources Optimization
- Agricultural Return Flow Simulation
- Flood Projection under Changing Climate Conditions
Links
Selected Publications Prior to Employment with DRI:
Li, K., Huang, G., Wang, S., Razavi, S., & Zhang, X. (2022). Development of a joint probabilistic rainfall-runoff model for high-to-extreme flow projections under changing climatic conditions. Water Resources Research, 58, e2021WR031557. https://doi.org/10.1029/2021WR031557
Li, K., Huang, G., Wang, S., Baetz, B., & Xu, W. (2022). A stepwise clustered hydrological model for addressing the temporal autocorrelation of daily streamflows in irrigated watersheds. Water Resources Research, 58, e2021WR031065. https://doi.org/10.1029/2021WR031065
Li, K., G. Huang, S. Wang, and S. Razavi (2022), Development of a physics-informed data-driven model for gaining insights into hydrological processes in irrigated watersheds, Journal of Hydrology, 613, 128323. https://doi.org/10.1016/j.jhydrol.2022.128323
Li, K., Huang, G., & Baetz, B. (2021). Development of a Wilks feature importance method with improved variable rankings for supporting hydrological inference and modelling. Hydrology and Earth System Sciences, 25(9), 4947-4966. https://doi.org/10.5194/hess-25-4947-2021
Li, K., Huang, G., Zhang, X., Lu, C., & Wang, S. (2021). Temporal-Spatial changes of monthly vegetation growth and their driving forces in the ancient Yellow River irrigation system, China. Journal of Contaminant Hydrology, 243, 103911. https://doi.org/10.1016/j.jconhyd.2021.103911
Li, K., Huang, G., & Wang, S. (2019). Market-based stochastic optimization of water resources systems for improving drought resilience and economic efficiency in arid regions. Journal of cleaner production, 233, 522-537. https://doi.org/10.1016/j.jclepro.2019.05.379
Keywords
Hydrological modeling, Machine learning, Water resources optimization, Model inferences