SMART Laboratory
Science & Mathematics of AI modeling for Research and TechnologySMART Lab Missions:
Science & Mathematics of AI modeling for Research and Technology (SMART) Lab established in 2022. The SMART Lab has three core missions: Education, Research & Technology
Education
With over 20 years of experience in teaching and curriculum development at both national and international levels, we design and deliver courses aimed at educating the next generation of scientists, engineers, and educators. We offer students the opportunity to engage in solo or team research projects, fostering increased interest and achievement in STEM fields.
Research
We design, develop, and implement diverse research projects that heavily utilize advanced coding and computational skills, including AI techniques, machine learning models, big data analysis, physical models, and simulations, spanning a wide range of interdisciplinary research areas.
Technology
We develop innovative software and technologies using cutting-edge techniques in computer science and engineering. Our commitment to fostering collaboration among industry, STEM education, and academia drives continuous progress and innovation.
Lab Director
Dr. Farnaz Emily Hosseinpour
Director, SMART Lab, DRI
Assoc. Director and Professor of ATMS Graduate Program, UNR/DRI
Assoc. Director of NV NASA Programs, NSHEE-mail: Farnaz@dri.edu
Phone: 775-673-7458
Office: CRVB # 152
Selected Awards

The SMART Lab’s Computer Science and Engineering Team has won the ‘Public Choice Award’ for their innovative capstone project at the 2024 Innovation Competition, held among the College of Engineering students at the University of Nevada, Reno. Congratulations to the team of students!

Ghazal Mehdizadeh successfully passed her Doctoral Comprehensive Exam in April 2024. Congratulations Ghazal!

Rahele Barjeste Vaezi successfully presented the results of our NASA project at the 2024 NV NASA EPSCoR Annual Meeting. Congratulations Rahele!

Dr. Hosseinpour has been honored with the 2023 Graduate Advisor of the Year Award at the Desert Research Institute (DRI) and the University of Nevada, Reno (UNR). In response to this recognition, Dr. Hosseinpour remarked, “This award is an inspiring reminder to continue my commitment to advising and mentoring our students, who represent the future generation of scientists, engineers, and educators in our community. I am deeply grateful for this honor and look forward to many more years of leading the SMART Lab and guiding the next generation of scholars and innovators at DRI and UNR.“
Lab Personnel
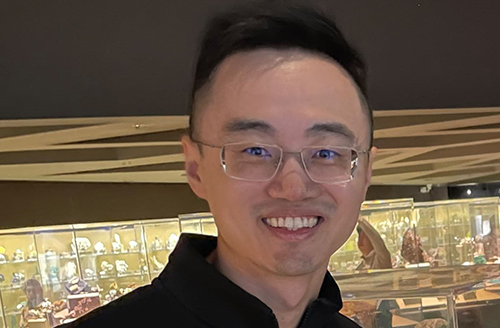
Kailong Li
Postdoctoral Researcher
Machine Learning Modeling
Email: kailong.li@dri.edu
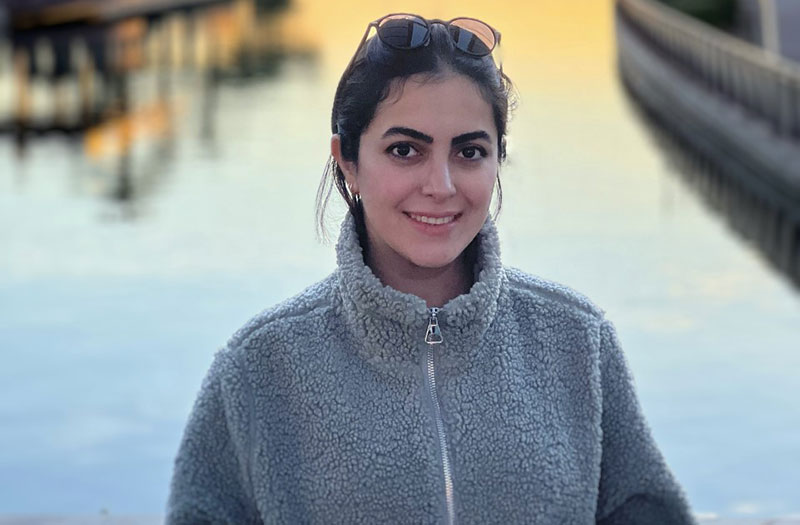
Ghazal Mehdizadeh
Ph.D. Student, Atmospheric Sciences
Graduate Research Assistant
E-mail: ghazal.mehdizadeh@dri.edu
View LinkedIn Profile
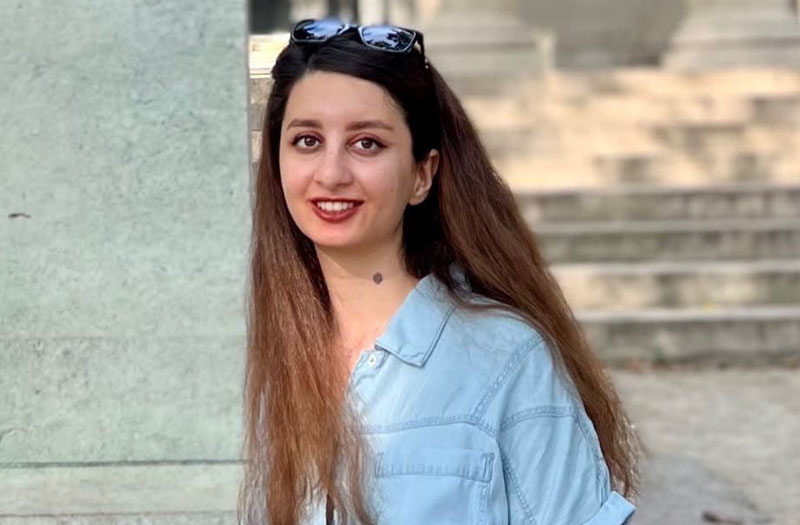
Rahele Barjeste Vaezi
Ph.D. Student, Atmospheric Sciences
Graduate Research and Teaching Assistant
E-mail: r.vaezi@nevada.unr.edu
View LinkedIn Profile
Former Students
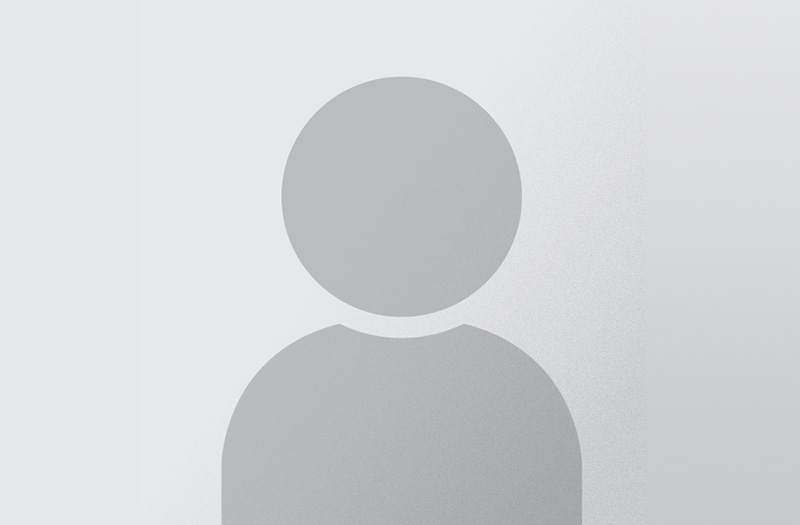
Richard Cao
Computer Science & Engineering Student
Email: richardcao@nevada.unr.edu

Cheng-Hsiang Chang
Atmospheric Sciences Ph.D. student
University of Nevada Reno
Email: chenghsiangc@nevada.unr.edu

Maryam Ghaed
Master's Student and Intern, Computer Sciences & Engineering
Email: mghaed@unr.edu
View LinkedIn Profile

Aydyant Khatri
Computer Science Master’s Student
Virginia Tech University
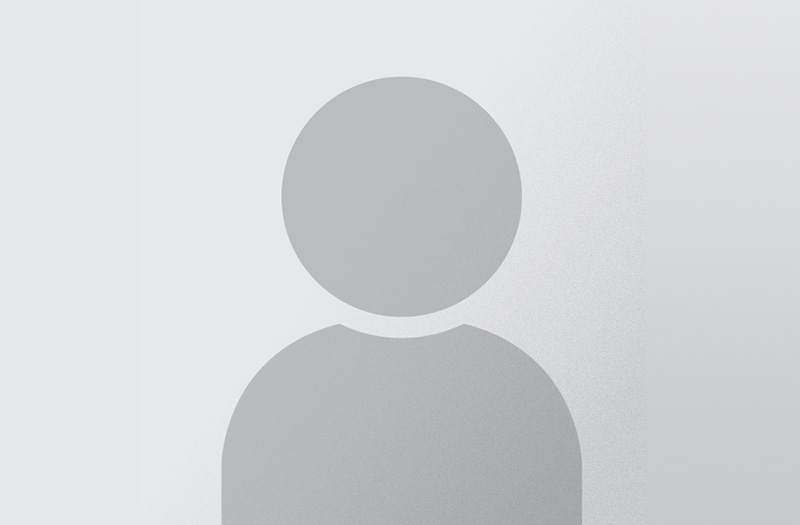
Jeremy Mamaril
Computer Science & Engineering Student
E-mail: jmamaril@nevada.unr.edu
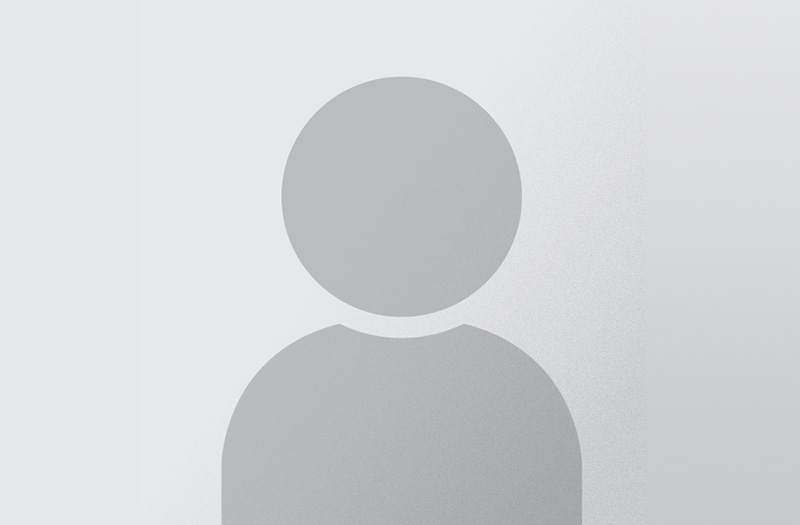
Mark Masala
Computer Science & Engineering Student
E-mail: markmarsala@nevada.unr.edu

Roya Salek Shahrezaie
Ph.D. Student and Intern, Computer Sciences & Engineering
E-mail: roya.salek@gmail.com
View LinkedIn Profile
Current Projects
Development of Smoke Transport Probability and Risk Interactive Map from Trajectories and Climatology Analysis of 2-km CANSAC-Reanalysis Database
PI: Dr. Farnaz Hosseinpour
Funded by: Department of Forestry and Fire Protection (CAL FIRE), Forest Health Program
Total award amount: $445,000.00
Project Description: This project generates high-resolution smoke transport probability for the 2km CANSAC reanalysis domain for use in prescribed fire planning and emergency response. Population, school, and hospital counts within each smoke transport region will be calculated for health risk management. Climatological fire weather metrics are generated and training workshops are held for stakeholders to improve the final product and demonstrate the intended use of the data for decision-making.
WRCC Disaster Relief Supplemental Appropriations (DRSA) for fire weather dataset activities
Co-PI: Dr. Farnaz Hosseinpour
Funded by: NOAA/National Centers for Environmental Information
Total award amount: $200,000.00
Project description: The NOAA National Centers for Environmental Information (NCEI)/Climate Science & Services Division (CSSD) contracts with the Regional Climate Centers (RCCs) to provide regional climate services. The six centers that comprise the RCC Program provide efficient, user-driven services including (but not limited to) the provision and development of sector- specific and value-added data products and services. The Disaster Relief Supplemental Appropriations (DRSA) Act of 2022 charged NOAA to improve wildfire research, prediction, detection, forecasting, monitoring, data management, and communication and engagement. Specifically, NCEI has been charged with using artificial intelligence (AI) / machine learning (ML) tools to determine techniques to extend relevant humidity and vegetation condition data back in time, particularly to account for instrumentation changes that currently disallow an apples-to-apples comparison of today’s fire weather information. This Additional Work Request (AWR) covers one task, with three sub-tasks, related to extending such datasets back in time by leveraging the Western Regional Climate Center’s (WRCC’s) existing expertise in fire weather and dataset development. The WRCC will perform the tasks and provide the deliverables. In accordance with the DRSA Act, the deliverables will be subsequently used to analyze the consequences of U.S. wildfires in calendar years 2020 and 2021. This work supports preparedness, seasonal assessment, and real-time understanding of fire weather conditions.
Estimating Background Ozone Using Data Fusion
Co-PI: Dr. Farnaz Hosseinpour
Funded by: Electric Power Research Institute
Total award amount: $70,000.00
Project Description: In the current regulatory context, the U.S. background (USB) ozone refers to ground-level ozone produced by anthropogenic pollution from emission sources outside the U.S., global natural emissions, and natural events such as wildfires or stratospheric intrusions. The USB ozone represents the theoretical minimum ozone concentration achievable through U.S. regulatory policy. As anthropogenic precursor emissions decrease nationally, the fraction of ozone that is USB may increase and under more stringent National Ambient Air Quality Standard (NAAQS) for ozone, the USB may cause exceedances of the NAAQS.
The four main sources of USB are stratospheric intrusions, biomass burning, international transport, and other natural emissions. Background ozone concentrations are higher in the West than in the East as a result of 1) a larger number of wildfires, 2) higher altitude sites in the West being more susceptible to impacts from stratospheric ozone intrusion, and 3) a greater impact from international transport. Since there are no measurements of background ozone, photochemical models are used to derive estimate of USB by conducting sensitivity simulations where anthropogenic emissions are zeroed out. Although the results from recent background ozone modeling studies are relatively consistent, differences and uncertainties in model inputs, processes, and chemical mechanisms remain.
Recently data fusion methods have been used in conjunction with photochemical models and observed ozone concentrations to reduce uncertainty in estimates of USB. We would apply such models to improve estimates of USB in different urban areas across the U.S. Our goal would be to optimize the least square regressions method at individual cities, as well as to test other methodologies to improve estimates of USB at those cities.
Obtained the 2016 air quality modeling platform from EPA and Conducted ozone season modeling for the whole U.S. for 2016: EPA has developed a revised version (Version 2) of the 2016 modeling platform that is available publicly. We obtained all the pre-merged emissions data as well as the meteorological data from EPA for this modeling platform. We conducted the base case modeling using the 2016 modeling platform input data obtained in Task 1 for the ozone season (April to September) using a 12-km horizontal grid, and repeated the ozone season simulation without the anthropogenic emissions to estimate modeled background ozone for the whole U.S. We selected 13 urban areas in the U.S. in consultation with EPRI project manager and conducted a model evaluation for the base case simulation in those locations.
Applied data fusion to improve estimates of background ozone: We applied the least square regression approach to fuse observed ozone and the modeled background ozone at the 13 urban areas and obtained improved estimates of background ozone at those locations. This required optimizing the regression parameters at each location. We applied several machine learning models to investigate how that methodology performs against the least square regression approach.
Modeling and Source Attribution of ozone in southeastern New Mexico
PI: Dr. Farnaz Hosseinpour
Funded by: New Mexico Environmental Department
Total award amount: $60,000.00
Project description: The New Mexico Air Quality Control Act requires the New Mexico Environment Department (NMED) to develop and adopt a plan, including regulations, to control emissions of oxides of nitrogen and volatile organic compounds to provide for the attainment and maintenance of the ozone NAAQS (National Ambient Air Quality Standard) when ozone concentrations are in excess of 95% of the ozone NAAQS (NM Stat § 74-2-5.3 ,2017). According to NMED – Ozone Attainment Initiative (OAI) program, only the Sunland Park area in southern New Mexico is currently classified as nonattainment. However, other counties are seeing increased ozone concentrations, with seven monitors exceeding 95% of the ozone NAAQS, two of which fall within Lea and Eddy Counties, southeastern New Mexico (SE-NM) (NMED, 2021; NMED-AQB, 2021). These counties are located in the furthest southwest corner of the state where oil and gas exploration activities in the Permian Basin are intense. Moreover, this region is adjacent to Mexico and the neighboring state of Texas, making it to be more complicated for the state of New Mexico to develop the most effective emission control strategies to reach the attainment of the standard. Therefore, it is important for federal/state and local authorities to rely on a comprehensive analysis of source attribution of New Mexico and non-New Mexico sources to air quality in this area when developing emission control strategies. In this study, we will assist NMED in quantifying source attribution to air quality in SE-NM by 1) Reviewing the current status and trend of air quality in the region using observations; 2) Quantifying source attribution of New Mexico versus non-New Mexico sources to the local air quality by leveraging source apportionment analysis performed by Ramboll and WESTAR (2021) in combination with our own back trajectories as well as source apportionment modeling targeting upwind individual high-emitting sources; 3) Providing technical advice to NMED based on the modeling findings to prepare petition 126 submitted to EPA for enforcing the “Good Neighbor Provision” if source attribution analysis identifies any emitter in the upwind state significantly affect air quality in SE-NM.
Smoke concentration predictions
Co-PI: Dr. Farnaz Hosseinpour
Funded by: Climate AI
Total award amount: $52,000.00
Project Description:
Smoke Climatology and Transport: A comprehensive climatological analysis has been conducted using the atmospheric variables (e.g., wind, temperature, moisture, etc.) from CANSAC historical data, available hourly for 1996 to present on the DRI server. The data analysis has been done over the D02 domain that covers the West Coast and parts of the central US with 6 km spatial resolution. This reanalysis product is the result of running the WRF model. In addition, to investigate the smoke climatology and transport, we applied historical MERRA-2 data with a spatial resolution of 0.5 X 0.65 degrees. For this purpose, we extracted the daily aerosol information and calculated PM2.5 and PM10 for the period of August to October 1996-2020. An essential aspect of MERRA-2 is the assimilation of bias-corrected aerosol optical depth and physical properties of aerosols from the various ground- and space-based remote sensing platforms. Previous studies showed that the MERRA-2 system provides the best estimate of the atmosphere state historically from the present day back to 1980. MERRA-2 aerosol reanalysis is based on the GEOS-5 Goddard Aerosol Assimilation System (GAAS), and the MERRA-2 aerosols are simulated with a radiatively coupled version of the Goddard Chemistry Aerosol Radiation and Transport (GOCART; Colarco et al., 2010) aerosol model. This model uses the assimilated meteorological fields of the Goddard Earth Observing System Data Assimilation System (GEOS DAS). Using the interactive GOCART aerosol module, the GAAS includes 15 aerosol tracers (dust, sea-salt, sulfate, black and organic carbon) driven by prescribed sea- surface temperature and sea-ice, daily volcanic, and biomass burning emissions, as well as high-resolution inventories of anthropogenic emission sources. Thus, MERRA-2 provides observationally constrained aerosol speciation determined from the GOCART model.
Developed smoke model: We applied the Bluesky framework to compare smoke concentration between Consume model and MERRA-2 as the initial step for predicting smoke over the receptor areas. Consume (Hollis et al., 2010) is a Bluesky model that produces emissions including PM2.5 and PM10 based on fire ecology and physics. We developed two primary smoke models, over various domains with various timescales (i.e., daily, weekly, and monthly). We trained the models over the period 2000-2019 to develop the predicting smoke model for the period 2020-2098. We applied ensemble machine learning algorithms (MLAs) to develop the smoke model to predict the PM2.5 and PM10 from the input variables. The machine learning methods we applied for this study include random forest (RF) (Breiman 2001), Gradient Boosting (GB) (Friedman 2001), AdaBoost (Freund and Shapire 1995), and Lasso (Tibshirani, 1996). All these produced similar results, while RF or GB provided the most stable and consistent outcomes. RF is an ensemble model used for both classification and regression by applying multiple decision trees as parallel. We used RF method for regression as the outcome variables are continuous and return the mean prediction of the individual trees. We also tested voting among RF and GB and stacking RF and GB, but the accuracy was not better than RF and GB alone; therefore, we used RF to develop and project to avoid the computation expense. This is also consistent with the previous studies which used RF to investigate smoke from wildfires.
Sierra Nevada Extreme Weather: A Novel Investigation of the Energetics of Atmospheric Rivers
PI: Dr. Farnaz Hosseinpour
Funded by: Nevada NASA EPSCoR Program
Total award amount: $38,000.00
Project description: Although Atmospheric Rivers(ARs) strongly influence both extreme precipitation and the global water cycle through their cumulative effects, the horizontal water vapor fluxes of ARs are poorly observed by the global atmospheric observing systems (Ralph et al., 2004). This study is motivated by the need to better predict extreme precipitation events along the west coast of California and the Sierra Nevada. Since weather data is highly nonlinear and follows irregular trends, we pursue advanced techniques that can be used to explore further dynamics of the ARs associated with extreme weather. By advancing the diagnoses of AR characteristics, this study aims to fill the gaps limiting past diagnoses of ARs. A key goal of this study is to explain in detail the energetic aspects of the ARs that can result in heavy rainfall over the Sierra Nevada. This study showed what combinations of the environmental structure are related to the growth and amplification of the eddy energetics related to the ARs that motivate extreme precipitation in the Sierra Nevada region. Using an ensemble of NASA datasets, a detection algorithm was developed and employed to identify ARs in eddy energy budget fields. We defined the thresholds of the energetic parameters, above which, ARs are likely to occur on the West Coast and the Sierra Mountains. In this manner, we provided an advanced diagnostic technique to detect the ARs through the exchanges of energy with background mean- circulations.
Completed Projects
A New Look into the Impacts of Dust Radiative Forcing on the Energetics of Tropical Easterly Waves
This research was provided by the start-up fund and the results are published in Hosseinpour and Wilcox (2023) and are presented at several conferences.
Saharan dust aerosols are often embedded in tropical easterly waves, also known as African easterly waves, and are transported thousands of kilometers across the tropical Atlantic Oceans, reaching the Caribbean Sea, Amazon Basin, and the eastern U.S. However, due to the complexity of the African and Atlantic climate dynamics, there is still a lack of understanding of how dust particles may influence the development of African easterly waves, which are coupled to deep convective systems over the tropical Atlantic Ocean and in some cases may seed the growth of tropical cyclones. We applied 22 years of daily satellite observations and MERRA-2 reanalysis to explore the relationships between dust in the Saharan air layer and the development of African easterly waves. Our findings show that dust aerosols are not merely transported by the African easterly jet and the the waves system, but also contribute to the changes in the eddy energetics of the waves.
The radiative forcing efficiency of dust in the atmosphere is estimated to be a warming of approximately 20 Wm-2 over the ocean and 35 Wm-2 over land. This diabatic heating of dust aerosols acts as an additional energy source to increase the growth of the waves. Our findings also show that dust outbreaks over the tropical Atlantic Ocean precede the development of baroclinic waves, which suggests that the dust radiative forcing can trigger the generation of the transient eddies in the system comprising the African Easterly Jet and African easterly waves.
Image Above: Composite 600-hPa 2-6-day filtered EKE (m2 s -2 2 ) values for the times corresponding to the upper 3 quartile aerosol radiative forcing minus the EKE values of the times corresponding to the lower quartile 4 aerosol radiative forcing over the OSAL domain (rectangle in Figure 2a). The calculations are conducted using the MERRA-2 reanalysis for JJA, 2000-2021. (b) Same as (a) but for 6-11-day filtered EKE (m2 s -2 5 ). (c) same as (a) but for the 2-6-day variance of zonal wind, 𝑢′ ̅̅̅2̅, (m2 s -2 6 ). (d) As in (a) but for 2-6-day the variance of meridional wind, 𝑣′ ̅̅̅2̅, (m2 s -2 ). (e) Same as (a) but for the 2-6-day filtered momentum fluxes, 𝑢 ′𝑣 ̅̅̅̅̅̅′ 7 , (m2 s -2 8 ).
Predictability of Wildfire Emissions and Transport in the Western US
Our wildfire research projects are funded by NOAA and USFS grants as well as ClimateAI, in which the results are reported in Hosseinpour and Brown (2021) and presented at several conferences (e.g. Hosseinpour et al., 2023).
Wildland fires in the Western U.S. cause significant damage to infrastructure, impact air quality, and increase greenhouse gases. Fire management and air quality agencies utilize smoke emissions data and forecasts to inform a variety of decisions. Since the beginning of COVID, a need was identified to develop new smoke assessment tools that can be integrated into a national Smoke-COVID dashboard developed by the USFS for operational use. Various research efforts around the country are demonstrating that smoke human health impacts, due to its impacts on respiratory and immune system functioning, is increasingly being exacerbated given COVID including increasing susceptibility and the rate of adverse outcomes (e.g., morbidity). Using various historical data (e.g., CANSAC data) from 1984 to 2019, Consume model and machine learning (ML) techniques, the seasonal-to-subseasonal predictions of smoke emissions was explored over California and Nevada. The essential inputs of the Consume model include Monitoring Trends and Burn Severity (MTBS) burned area, GridMET 1000-hr, duff, litter fuel moistures (FMs), and Fuel Characteristic Classification System (FCCS) fuelbed data. The historical datasets are applied based on additional meteorological variables such as maximum temperature (Tmax), maximum wind, vapor pressure deficit (VPD), and drought indicators such as the Standardized Precipitation Index (SPI). These data are compiled and grouped into five vegetation categories: broadband forest, conifer forest, mixed forest, grassland/savanna, and shrubland such that assessments can be undertaken for the vegetation type. The emissions model outputs are then examined for statistical relationships that can be broadly used on a monthly to seasonal scale for determining potential seasonal emissions predictions in conjunction with climate outlooks or model forecasts. The results of applying multiple ML algorithms show that Random Forest performs the best, compared to other ML algorithms. Also, burned area, daily maximum wind speed, and daily maximum temperature are the most important input variables in determining smoke emissions by ML techniques.
Image Above: Fire-related variables for 1984-2019 in Northern California. Climatological JAS averages are shown for variables. The exception is the number of fires per pixel.
Using Machine Learning Methods in Aerosol-Cloud Interactions and Air Quality
We conducted various studies to advance our understanding of aerosol-cloud interactions impacts on climate (Hosseinpour et al., 2023), fire impacts on clouds (Chang and Hosseinpour, 2023), and the impacts of meteorological conditions on particulate matters (PMs) (Khatri and Hosseinpour, 2022).
Air pollutions including aerosols have a critical influence on regional and global climate through their impacts on radiation, clouds, hydrological cycle, and atmospheric circulation. These interactions include the impacts of aerosols on climate directly via absorbing or scattering solar and/or terrestrial radiations and indirectly by serving as cloud condensation nuclei (CCN) and ice nuclei (IN).
The first study mentioned above investigated a record-breaking Saharan dust storm, known as the “Godzilla” extreme event, which caused a considerable trans-Atlantic transport of Saharan dust and poor air quality conditions over the Eastern US in Summer 2020. We used various NASA satellite and reanalysis products and conduct statistical analysis including principal components analysis (PCA) and showed that the near-surface temperature and specific humidity are essential for low-level cloud cover over the tropical Atlantic Ocean. A mechanism was provided to explain the dust-induced enhancement of clouds through the semi-direct aerosol effect: dust aerosols above the inversion layer cause the warming and drying in the dust layer and moistening of the air below the dust layer that favors cloud formation.
The second study used 20 years of reanalysis and satellite observations to investigate the importance of cloud-controlling factors and aerosols on the cloud variables in two stratocumulus deck regions. The explainable MLAs showed that in addition to meteorological conditions, aerosols are crucial in determining cloud fraction and cloud effective radius.
In the third study, we use MLAs to explore the prediction of PM2.5 and PM10 during the 23 wildfire seasons in California and Nevada. Multiple neural network techniques were applied, and various metrics (R-squared and RMSE) were calculated. The results showed the applications of ML method in predicting air pollution.
Image Above: Upper panels: Climatoligcal mean of 75th percentile of AOD from MERRA2 for the Northeast Pacific (left) and the Northeast Atlantic. Lower panels: Model evaluations of low-level cloud fraction (CF), cloud effective radius (CER), and cloud top temperature (CTT) for Northeast Pacific (left) and the Northeast Atlantic. Blue bars show MLA without considering aerosols and orange bars show MLA with considering aerosols.
Impact of Dust on Climate Dynamics
This research was funded by NASA and the results have been published in Hosseinpour (2017) and Hosseinpour and Wilcox (2014), and presented at multiple conferences.
Mechanistic relationships exist between the variability of dust in the oceanic Saharan air layer (OSAL) and transient changes in the dynamics of Western Africa and the tropical Atlantic Ocean. This study provides evidence of possible interactions between dust in the OSAL region and the African easterly jet–African easterly wave (AEJ–AEW) system in the climatology of boreal summer when easterly wave activity peaks. Synoptic-scale changes in instability and precipitation in the African/Atlantic intertropical convergence zone are correlated with enhanced aerosol optical depth (AOD) in the OSAL region in response to anomalous 3D overturning circulations and upstream/downstream thermal anomalies above and below the mean-AEJ level. Upstream and downstream anomalies are referred to the daily thermal/dynamical changes over the West African monsoon region and the Eastern Atlantic Ocean, respectively. We hypothesize that AOD in the OSAL is positively correlated with the downstream AEWs and negatively correlated with the upstream waves from a climatological perspective. The similarity between the 3D pattern of thermal/dynamical anomalies correlated with dust outbreaks and those of AEWs provides a mechanism for dust radiative heating in the atmosphere to reinforce AEW activity. We proposed that the interactions of OSAL dust with regional climate mainly occur through the coupling of dust with the AEWs.
Image above: (a) Vertical-longitudinal cross-section of correlation (shaded) between MODIS AOD in the OSAL and temperature profile, meridionally averaged over the AOD domain, for climatology of boreal summer (JJA) from 2000 to 2012. (b) Long-term mean (shaded) of MODIS AOD and the rectangle is loading AOD domain representing OSAL. (c) Horizontal correlation (shaded) between temperature (K) at 850 hPa and AOD in the loaning domain; contours represent long-term mean of temperature at 850 hPa.
CONTACT
Farnaz Hosseinpour, Ph.D.
Lab Director
Farnaz.Hosseinpour@dri.edu
LAB LOCATION
Desert Research Institute
2215 Raggio Parkway
Reno, NV 89512